2024-05-11 by Admin
The Critical Role of Data Quality in AI: Ensuring Accurate Model Outputs
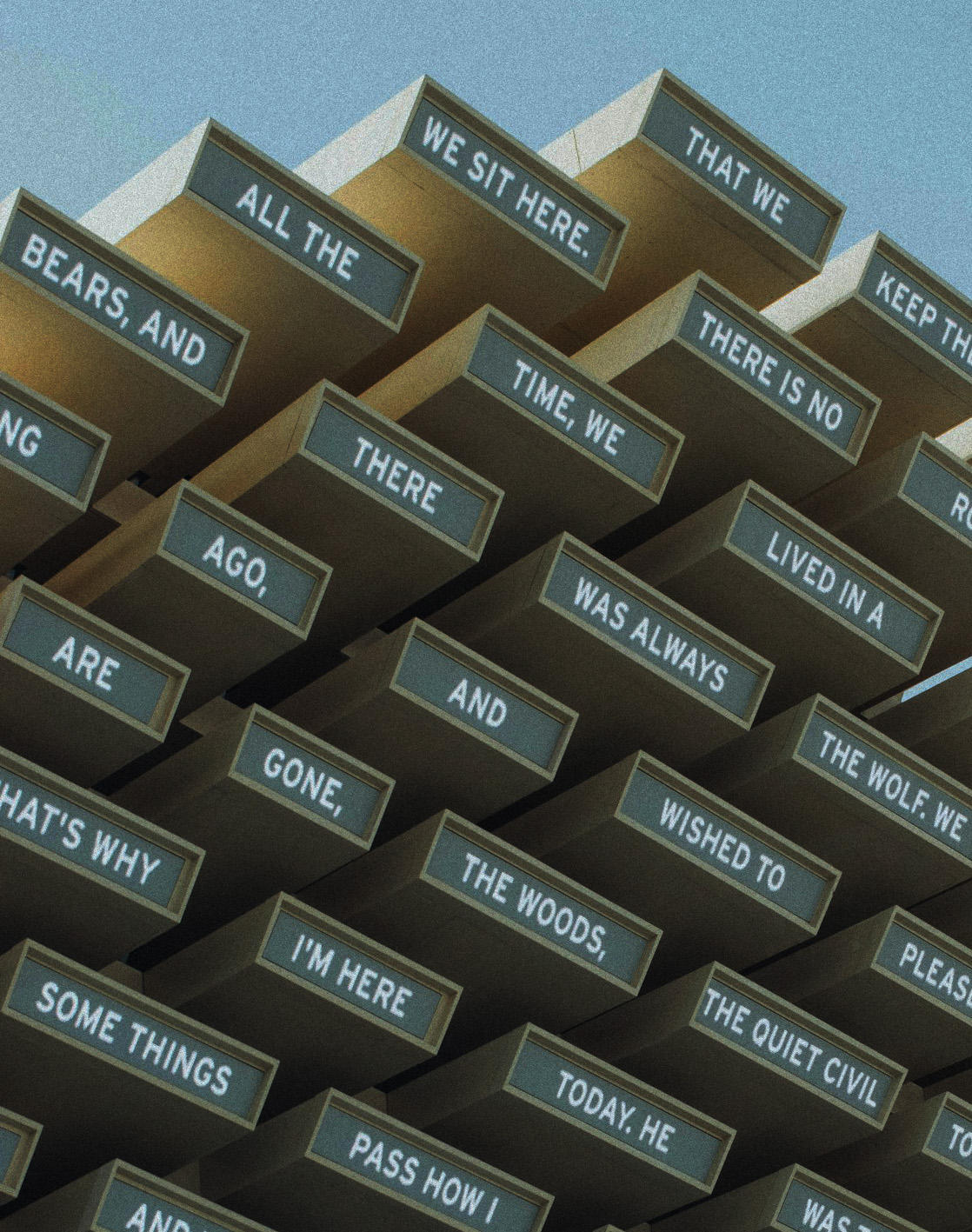
In the realm of artificial intelligence (AI), data is the lifeblood that fuels the engines of innovation. From powering recommendation systems to driving autonomous vehicles, the quality of data directly impacts the performance and reliability of AI models. However, the old adage "garbage in, garbage out" holds true in the AI landscape perhaps more than anywhere else. In this article, we'll explore the profound importance of data quality in AI and how overlooking this crucial aspect can lead to disastrous consequences.
Imagine constructing a magnificent skyscraper on a shaky foundation – it's bound to collapse sooner or later. Similarly, AI models built upon poor-quality data are inherently flawed, leading to unreliable predictions and erroneous outcomes. Whether it's incomplete, inaccurate, or biased data, the repercussions of subpar data quality are manifold and can reverberate across various domains.
First and foremost, bad data can distort the insights derived from AI models, leading to flawed decision-making. Consider a healthcare AI tasked with diagnosing diseases based on patient data. If the training dataset contains inconsistent or erroneous medical records, the model's predictions will be unreliable, potentially endangering patients' lives. In such critical scenarios, the ramifications of data quality lapses are not just theoretical but have real-life consequences.
Moreover, the insidious nature of biased data poses a significant threat to the ethical integrity of AI systems. Biases inherent in the training data – whether stemming from historical disparities, societal prejudices, or algorithmic biases – can perpetuate and exacerbate inequalities. For instance, biased data in hiring algorithms can inadvertently discriminate against certain demographic groups, perpetuating systemic biases rather than mitigating them.
Furthermore, the proliferation of AI across various sectors underscores the need for data quality standards and governance mechanisms. In fields like finance, autonomous driving, and criminal justice, erroneous AI predictions can have far-reaching implications, affecting economic stability, public safety, and individual rights. Therefore, ensuring data quality isn't just a matter of technological prowess but a moral imperative and a regulatory necessity.
Addressing the challenges of data quality requires a multi-faceted approach encompassing data collection, preprocessing, and ongoing monitoring. Rigorous data validation techniques, such as outlier detection and anomaly detection, can help identify and rectify discrepancies in the dataset. Additionally, employing diverse and representative datasets can mitigate the risk of biases creeping into AI models.
Furthermore, transparency and accountability are paramount in safeguarding against the pitfalls of bad data. Organizations must adopt transparent AI practices, including documenting data sources, model architectures, and decision-making processes. Moreover, establishing mechanisms for ongoing monitoring and auditing of AI systems can help detect and rectify data quality issues before they escalate.
In conclusion, the importance of data quality in AI cannot be overstated. As AI continues to permeate every aspect of our lives, ensuring the reliability and integrity of AI models is imperative. By prioritizing data quality standards, embracing transparency, and fostering a culture of responsible AI development, we can harness the transformative potential of AI while mitigating its inherent risks. After all, in the quest for technological advancement, the quality of our data determines the caliber of our achievements.